Fine tuning Deep Neural Networks in Continuous Learning Scenarios
Fine-tuning neural networks
In this post, we'll discuss what fine-tuning is and how we can take advantage of it when building and training our own artificial neural networks.
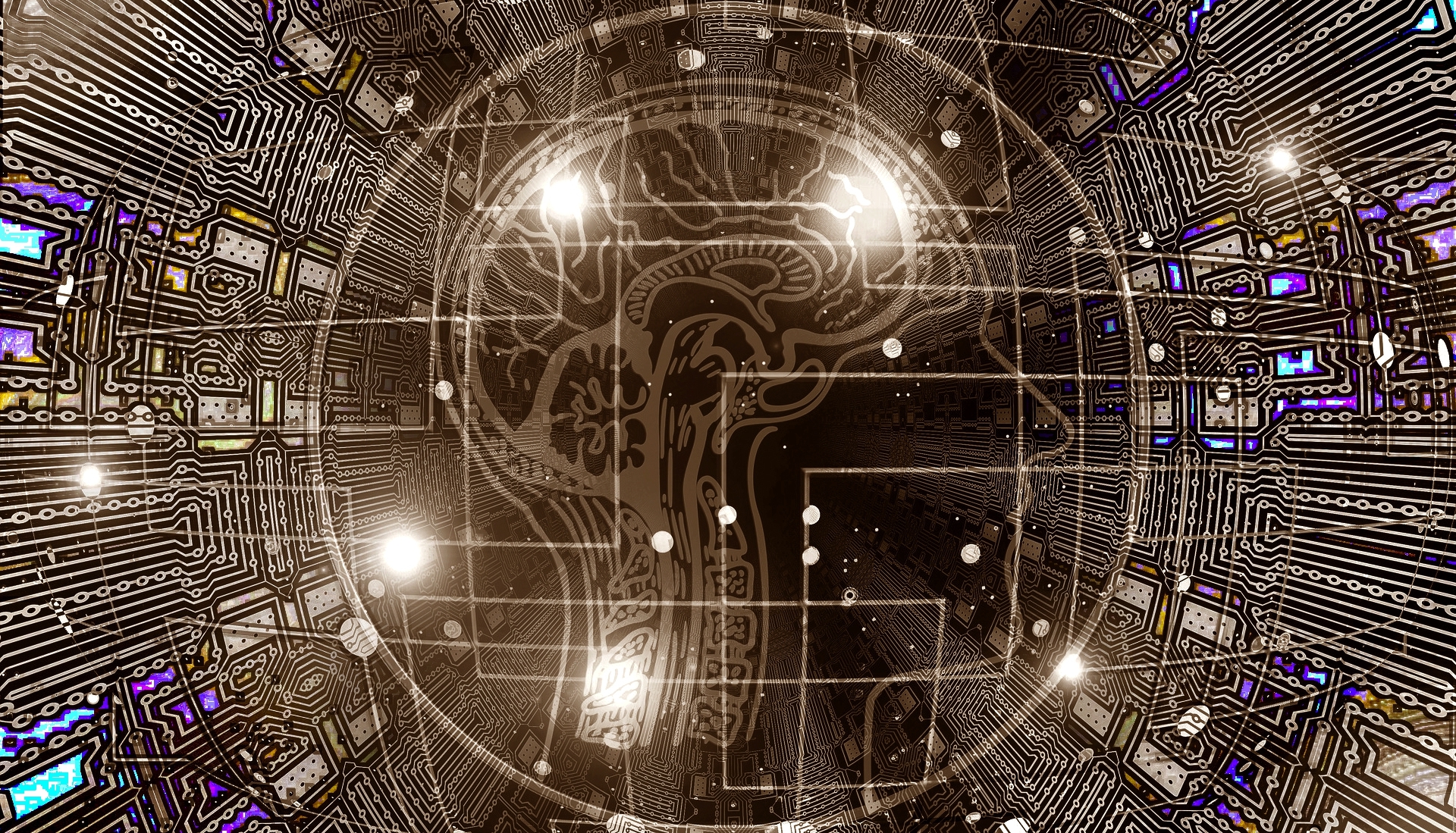
Introducing fine-tuning and transfer learning
Fine-tuning is very closely linked with the term transfer learning. Transfer learning occurs when we use knowledge that was gained from solving one problem and apply it to a new but related problem.
Transfer learning occurs when we use knowledge that was gained from solving one problem and apply it to a new but related problem.
For example, knowledge gained from learning to recognize cars could be applied in a problem of recognizing trucks.
Fine-tuning is a way of applying or utilizing transfer learning. Specifically, fine-tuning is a process that takes a model that has already been trained for one given task and then tunes or tweaks the model to make it perform a second similar task.
Why use fine-tuning?
Assuming the original task is similar to the new task, using an artificial neural network that has already been designed and trained allows us to take advantage of what the model has already learned without having to develop it from scratch.
When building a model from scratch, we usually must try many approaches through trial-and-error.
For example, we have to choose how many layers we're using, what types of layers we're using, what order to put the layers in, how many nodes to include in each layer, decide how much regularization to use, what to set our learning rate as, etc.
- Number of layers
- Types of layers
- Order of layers
- Number of nodes in each layer
- How much regularization to use
- Learning rate
Building and validating our model can be a huge task in its own right, depending on what data we're training it on.
This is what makes the fine-tuning approach so attractive. If we can find a trained model that already does one task well, and that task is similar to ours in at least some remote way, then we can take advantage of everything the model has already learned and apply it to our specific task.
Now, of course, if the two tasks are different, then there will be some information that the model has learned that may not apply to our new task, or there may be new information that the model needs to learn from the data regarding the new task that wasn't learned from the previous task.
For example, a model trained on cars is not going to have ever seen a truck bed, so this feature is something new the model would have to learn about. However, think about everything our model for recognizing trucks could use from the model that was originally trained on cars.
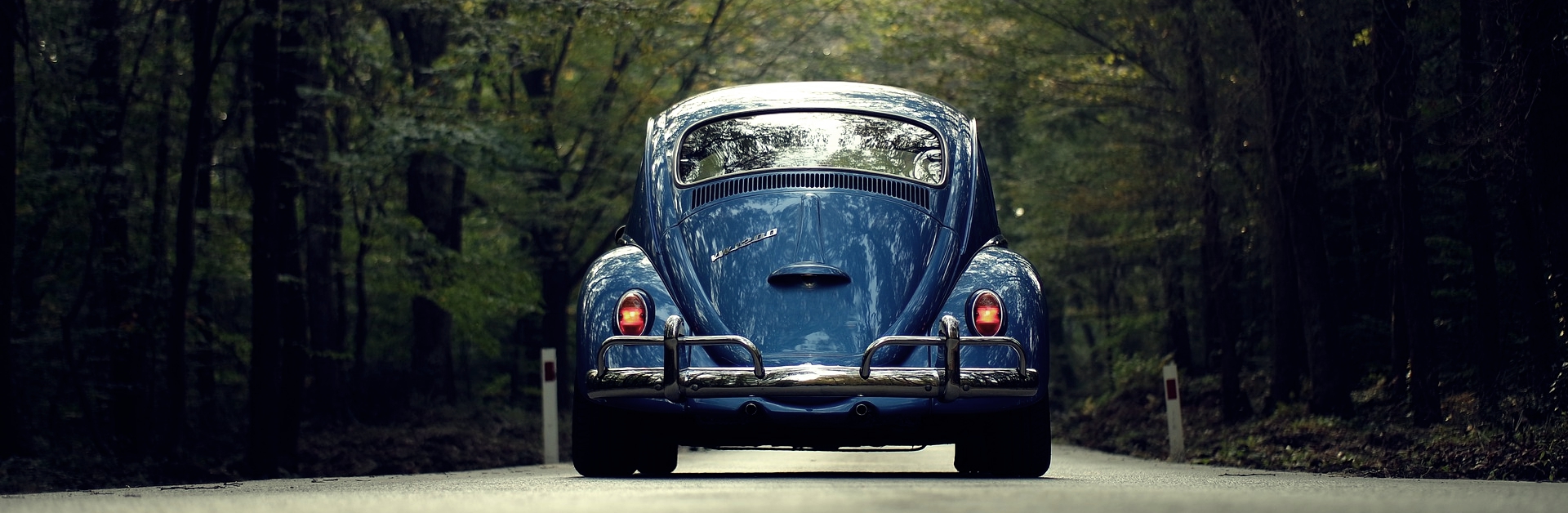
This already trained model has learned to understand edges and shapes and textures and more objectively, head lights, door handles, windshields, tires, etc. All of these learned features are definitely things we could benefit from in our new model for classifying trucks.
So this sounds fantastic, right, but how do we actually technically implement this?
How to fine-tune
Going back to the example we just mentioned, if we have a model that has already been trained to recognize cars and we want to fine-tune this model to recognize trucks, we can first import our original model that was used on the cars problem.
For simplicity purposes, let's say we remove the last layer of this model. The last layer would have previously been classifying whether an image was a car or not. After removing this, we want to add a new layer back that's purpose is to classify whether an image is a truck or not.
In some problems, we may want to remove more than just the last single layer, and we may want to add more than just one layer. This will depend on how similar the task is for each of the models.
Layers at the end of our model may have learned features that are very specific to the original task, where as layers at the start of the model usually learn more general features like edges, shapes, and textures.
After we've modified the structure of the existing model, we then want to freeze the layers in our new model that came from the original model.
Freezing weights
By freezing, we mean that we don't want the weights for these layers to update whenever we train the model on our new data for our new task. We want to keep all of these weights the same as they were after being trained on the original task. We only want the weights in our new or modified layers to be updating.
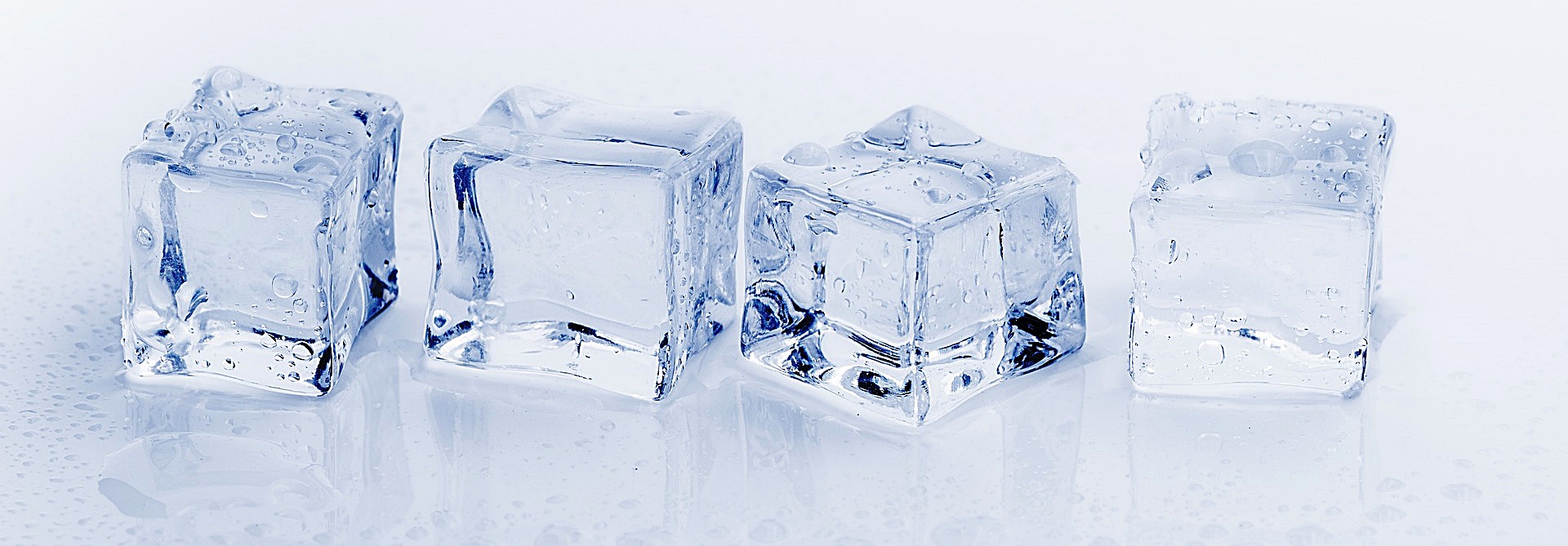
After we do this, all that's left is just to train the model on our new data. Again, during this training process, the weights from all the layers we kept from our original model will stay the same, and only the weights in our new layers will be updating.
Wrapping up
At this point, we should have a good idea about what fine-tuning a neural network is and why it's useful. To see fine-tuning in action, be sure to check out the Keras series that shows how to build a fine tuned model, how to train the model, and how to use the model to predict on new data. Definitely check those out if you want to see the technical implementation of fine-tuning written in code. I'll see you in the next one!
Source: https://deeplizard.com/learn/video/5T-iXNNiwIs
0 Response to "Fine tuning Deep Neural Networks in Continuous Learning Scenarios"
Postar um comentário